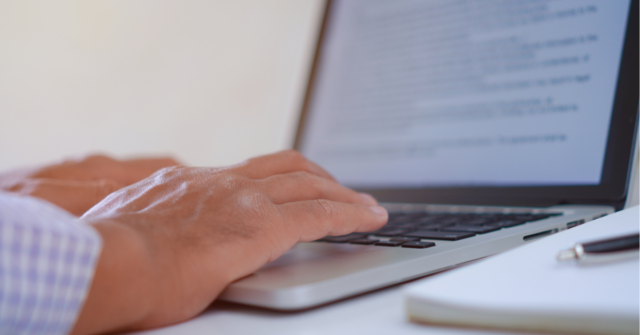
Marketers love data. Since the early days of Google Analytics, marketers have used data to take action and improve performance. Unfortunately, marketers now have access to enough data that they’re drowning in it. The need for metrics and the rise of available AI applications in the MarTech landscape have increased a marketer’s demand for data that is more easily actionable.
B2B marketers seek to make data actionable by extracting insights in three primary ways.
- Determining the right accounts to target.
- Personalizing and identifying appropriate content depending on where these target accounts are in their buyers’ journey.
- Measuring ROI from executed campaigns.
Marketers value these insights because they use them to run media campaigns that have the highest possible ROI based on hypertargeting and customization. They usually use recommendations or individual research to achieve both hypertargeting and customization and they usually must iterate to achieve the ROI they need.
The ML Data Cloud powers these insights with cutting-edge architecture. It also empowers Madison Logic’s solutions across multiple devices and media channels. This proprietary platform brings together data from varied first, second and third-party systems to create a best-in-class “Data Management Platform” for the B2B industry.
ML Data Cloud Benefits
Madison Logic’s solutions make it easy for marketers to target audiences, customize content and measure campaign effectiveness. When this process is orchestrated dynamically and with agility across all stages of the funnel, you’re implementing a multi-channel ABM strategy. In order to support this larger goal, the ML Data Cloud was built from ground up to provide the following:
Hypertargeting
Using data to define and narrow audiences with a wide range of filters. Some of these filters are:
-
- Firmographic: allowing users to narrow down by company’s revenue size, employee size, geo-location, industry, etc.
- Intent: helping marketers to filter by topics of interest that individuals in the company are currently searching for or engaging with.
- Audience: enabling one to whittle down individuals within companies by job titles, professional groups, departments, etc.
- Technographic: providing the capability to focus only on those companies that contain, or not contain, certain products, specific age of the product, end-of-life of assets, etc.
Customization
Utilizing data to choose and apply different content for different target audiences across different stages of the funnel.
Measurement
Obtaining data insights into campaign effectiveness measured not merely against engagement, but also against volume, velocity and value of actual conversions. Marketers can view these engagements as:
-
-
- Leads, Impressions, Exposure time: Sliced by one or more attributes of program, company size, revenue, country, region, job-title, assets, target account, week, and industry
- Site Visits: By one or more of program, target account, and week
- Aggregates: Such as top ten accounts targeted, bottom ten, and average broken down by impressions, exposure time, site visits, and number of leads
- Funnel Metrics: Pipeline and revenue attribution, such as changes in the number of conversions (volume), changes in the size of conversions (value), and changes in the speed of conversions (velocity).
-
What Is the ML Data Cloud?
Residing entirely on AWS, ML Data Cloud is a high performance, cloud-scalable, and GDPR compliant system providing privacy, security and multi-tenancy. Thanks to the adoption of this modern microservices architecture, our data cloud can perform at scale. Speed is crucial to ensuring that marketers can change hyper-targeting, customizations and measurements dynamically in mid-flight or mid-campaign. Furthermore, the ML Data Cloud is also the repository for Machine Learning and AI-based pacing, categorized scoring of campaign efficiency, and recommendations for hyper-targeting and customization.
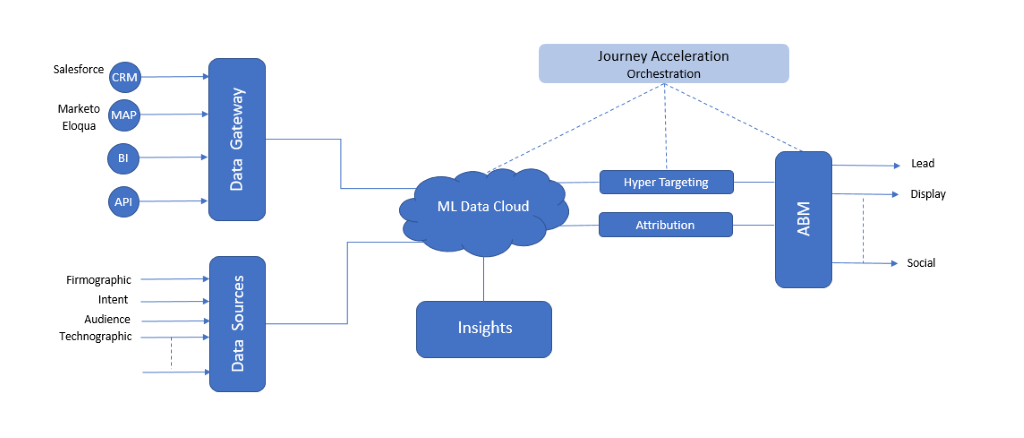
How ML Data Cloud Works
The Data Gateway allows for easy import of first-party data and export of necessary engagement metrics to various client CRM and MAP systems.
- Data pipelines bring in data from the Data Gateway and Data Sources and lands them in the ML Data Cloud (refer fig 1). The ML Data Cloud brings together 22 (and increasing) data sources across Firmographic, Intent, Audience, Technographic, engagement, public, first party and other data providers
- The ML Data Cloud then performs the necessary cleansing and enriching. This data is cleansed and enriched daily and is instrumental in creating Madison Logic’s proprietary data.
- Once Data is transformed and merged across the varied data sources it becomes usable for hyper-targeting and enabling ABM campaigns.
More About the Data:
Besides the 22+ data sources across Firmographic, Intent, Audience, Technographic, engagement, public, first party and other data providers, marketers can choose from more than 12 million companies across all company sizes, 40 verticals, and all regions around the globe. By virtue of tracking more than 15+ Billion B2B interactions monthly, these companies may also be filtered by over 5,700 intent topics, 9,000 installed products, 5000 vendors, 11 professional groups, 8 decision makers and thousands of job titles. Furthermore, audiences may be targeted over all device types for both lead generation and display advertising. The ML Data Cloud reaches 350+ million devices across the globe.
Conclusion
We want to make your ABM campaigns go from good to great! The powerful engine that is the ML Data Cloud fuels our solutions, enables Journey Acceleration, and ultimately enables marketers to convert their best accounts faster.
If you would like to learn more about how the ML Data Cloud can help accelerate your ABM Program, please contact us.